Multiple Kernel Learning for Dimensionality Reduction
IEEE Transactions on Pattern Analysis and Machine Intelligence 33 (2011): 1147-1160.
Yen-Yu Lin, Tyng-Luh Liu, and Chiou-Shann Fuh
- Institute of Information Science, Academia Sinica
In solving computer vision problems, adopting multiple descriptors to more precisely characterize the data has been a feasible way for improving performance. The resulting data representations are typically high dimensional and assume diverse forms. Thus finding a way to transform them into a unified space of lower dimension generally facilitates the underlying tasks, such as object recognition or clustering. To this end, the proposed approach (termed as MKL-DR) generalizes the framework of multiple kernel learning for dimensionality reduction, and introduces a new class of applications/techniques to address not only the supervised learning problems but also the unsupervised and semisupervised ones.
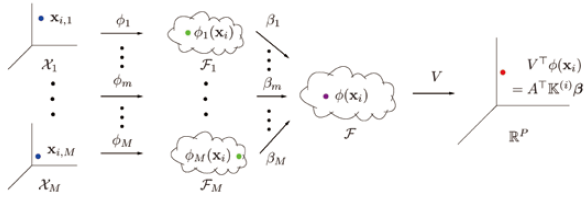